We can only believe the statistics that...
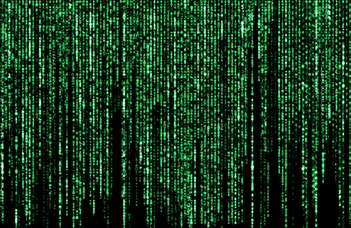
Although it is not true that Churchill himself said it, the wording still spreads like a verb. "I only believe the statistics I have forged myself" Although scientific researches hardly intend to falsify, it may be too tempting to choose an analytical methodology that seems suitable for verifying the research hypothesis. Therefore, Balázs Aczél and co-authors argue that:
We should only believe the statistical analysis that several research groups have proven by several methods.
Eric-Jan Wagenmakers, Alexandra Sarafoglou and Balázs Aczél, experts in research methodology and metascientific approach, have the attention to an anomaly around scientific predictions about the reproductive rate of COVID-19 infections led to the problem. They have noticed that independent groups of data analysts working with well-established statistical methods in principle
from the data available on the coronavirus have sometimes come to opposite conclusions.
These analyses examined the non-incidental question of whether the epidemic will be receding or spreading in the coming period. They all tried to calculate the so-called reproduction rate of the virus (i.e. R). However, while some groups predicted a value below 1, i.e. a decrease in the number of infections, others predicted a value above 1, i.e. the accelerating spread of the virus - although, in the opinion of the authors, in principle, epidemiologists could not have reached completely different conclusions from almost identical data.
Although one of the important criteria of science is still refutability, i.e. every analysis, every publication can lead to another, in which competing research teams can even refute the conclusions of their predecessors by repeating studies and analyses. According to Balázs Aczél and his co-authors, this follow-up control is not always enough. The rebuttal often arrives too late and often receives less publicity than the original result, i.e. even
erroneous conclusions can also keep themselves in the circle of scientific public opinion for a long time.
Therefore, the authors urge that the editors of scientific journals require that the calculations be checked by several methods when the articles are submitted; independent analytical teams should be conducted by separate analytical groups.
There is a lot of known criticism of the proposal, as multiple data analysis significantly pushes the publication date and makes research projects more expensive, while it is by no means worth the money and energy spent because, in many cases, the models and calculations of researchers prove to be correct, no matter how many times the analyses are run. At the same time, there are still several fields working with a high number of data; thus, it is common practice in high-energy particle physics or climate models to consciously test statistical models and examine the role of individual variables thoroughly. These disciplines can therefore serve as examples for other less data-oriented disciplines in their traditions.
The authors argue that the systematic introduction of multiple data analyses would strengthen public trust in science, especially in cases where research results directly impact society. Without it, there is a risk that the main character of the film "The Matrix" will be defeated.
We are going to act like Neo:
We choose the blue pill so that we can believe what we want to and not have to face reality.
You can read the full article here:
One statistical analysis must not rule them all
Nature, 19 May 2022.